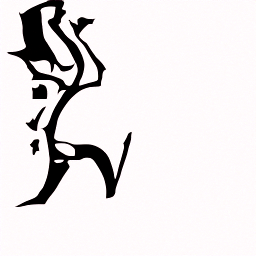
- Generative Projects
- Clip Guided Diffusion Experiments
- Tips and Tricks for TPUs
- Training v-diffusion-pytorch on TPUs
- Generating Python Code with Transformers
- Danbooru Utility
- Problem Solving with Deep Q-Learning at IEEE RoboResearch
- Deep Learning Talk at the Triangle IEEE Robotics and Automation group
- Why has someone been paying 100x market price for GPU instances?
Generative Projects
Posted some projects.
Clip Guided Diffusion Experiments
An overview of some experiments I ran on tpus (thanks to TPU Research Cloud) I'll be writing up details shortly.
Drawing Text
Can a generative model learn to render text directly? We could render a website with style and typography smoothly controllable by moving through the latent space, or smartly fix up a blurry image with text. Conversely we could learn about the edges in the capability of current generative models with a minimal example.
Font Generation Examples
Text Generation Examples
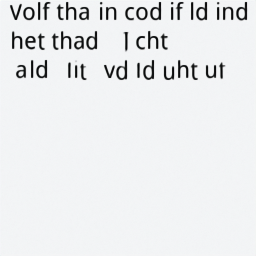
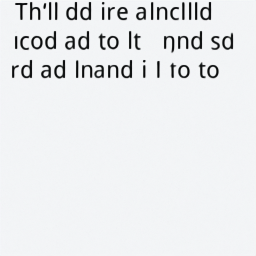
Model checkpoints:
Generating Sidewalk Delivery Robot Training Data
I trained a generative model on camera images from a sidewalk delivery robot to match text descriptions of the control input.
Sim to real and is a popular technique, particularly for reinforcement learning. Can generative models be used to create meaningful training data for real world robotics? Conversely can we use generative models to understand what the model is actually acting on because it will create examples for us?
Driving Instruction To Scene Example:
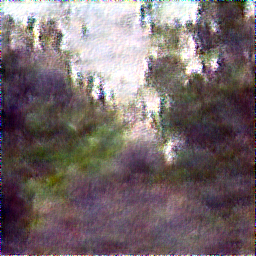
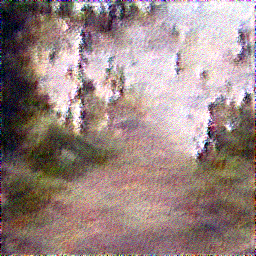
Model checkpoint: sidewalk robot image from instructions
Danbooru Manga Style Generation
Learn to generate using the tags and ratings in gwern's danbooru202x dataset.
Generate Emoji
Can a model learn to generate emojis based on the semantics of the text?
Tips and Tricks for TPUs
Some free form thoughts and recommendations for working with Tpu VM's, particularly training v-diffusion-pytorch. Thanks to TPU Research Cloud I had access to substantial compute (a lot more than I was expecting), giving me the opportunity to use cutting edge models and work on some old projects I had to give up on for lack of compute.
TRC Thoughts
Going into this I had the goal I would efficiently use all the tpus. Coordinating experiments on 4-5 tpu v3-8 took some practice, and following all the training run results, cutting off experiments when they failed, fixing bugs, and implementing tweaks and features took substantial work. I also ended up doing manual hyperparameter optimization that should have been scheduled. The libraries and tools I was used to working with (torch and Julia) have tpu support, but also have substantial issues (fatal in the case of Julia) and sharp edges. My logging situation was complicated by wandb errors on multiple cores. It took time to get torch_xla running properly. I never had a chance to run ray to train across tpus. I had visions of training on 100 preemptible tpus with automatic checkpoint syncing and restarting. This would be beautiful, but would also take time to get running. Nonetheless I was able to run models much larger than possible for me otherwise, train models overnight instead of in weeks, and run many parallel experiments. I also ended up with a better training setup to make using such compute easier in the future.
Tools and Libraries
Some thoughts on tools I'd recommend. I was still working with pretty simple tools, primarily running shell scripts to install everything, and starting the runs over ssh. I certainly could go further with more sophisticated tools and automation, but those have a cost as well.
Tmux
Tmux is a pain, but I don't know of a better alternative, because you do need a persistent ssh for long runs.
The default Tmux experience is impossible, but you can make it work with the config I posted in cloud setup scripts.
Biggest annoyance is that you can't copy to local clipboard, and the fixes are a massive headache.
A possible alternative Eternal Terminal doesn't seem to work.
Wandb
Having the web interface is really nice, particularly since getting tensorboard syncing across multiple vms would be difficult.
The attempts to make it easy to recreate your run exactly by providing git info is also quite nice.
Saving the system usage helpful.
I added logging of torch_xla metrics to help with performance profiling if a run is extra slow.
Note that wandb does not play well with multiple cores, hence the use of wandb service experiment.
It is still rather broken, and cannot log output or commands, so I added a basic command logger that save to command to config.
Code saving doesn't work.
init config saving doesn't work.
wandb.init()
andwandb.config.update()
do not work. If you want to add something to the config you need to set it manually with
wandb.config.yourvalue = 2345
Storage, Google drive
Using a cloud bucket or other storage option for storage and syncing is a more scalable method, but for small teams just using google drive works.
Data egress costs can be high, so using rsync or syncthing your checkpoints around can get expensive.
Mount your gdrive with fuse, and use it for syncing checkpoints and datasets to your vms.
You should probably also start your vms with a larger disk, so you don't have to worry about losing a run to lack of space, but that doesn't solve syncing.
Pytorch Lightning + Torch XLA
torch_xla has a reputation for being tricky, and it lived up to that reputation.
The setup is finicky, so I'd recommend following how I did it with cloud setup scripts.
The community was helpful. When I posted an issue based on
CompileTime too slow: longest instance took 14m30s ... Please open a Github issue with the graph dump for our team to optimize.
I received a swift and helpful response.All of the torch_xla colab examples are broken currently. Apparently torch_xla is not compatible with colab atm.
https://github.com/pytorch/xla/issues/3186
@cajoek It is related to colab's base os(I think it is ubuntu 18.02). PyTorch/Xla has moved its build to debian 10(to fix a different build issue) which has a newer version of GLIBC and incompatible with ubuntu. While colab team is working on upgrading their base os to a newer version and we are trying to build pt/xla on debian 9 too, both of these efforts goes pretty slowly.
Pytorch Lightning has a lot of convenience built in. This can be a bit tricky to decode when it goes wrong, but its hard to beat for that fiddling with training stage.
Julia
I really wanted to get Julia running on the tpus, particularly because I have a program synthesis project in Julia I want a lot more compute for.
XLA.jl is currently on hold. Probably until some compiler improvements arrive.
Pycalling Jax functions would probably work as long as its not too fancy.
Unfortunately Julia crashes with "free(): invalid pointer" or "double free or corruption (out)"
See this github issue I posted for details: https://github.com/JuliaLang/julia/issues/44242
Hopefully we can figure out what was going on here, but I didn't have much time to do so.
Jax
This might be the golden path for TPUs.
Sadly my old projects were not compatible.
If you are writing something lower level this seems like the way to go.
TPU difficulties
You are supposed to be able to stop a tpu vm and restart it without the ip address changing, but this is not always true. In one case a "maintenance event" swapped the ip addresses on two of my vms. This was rather confusing.
$gcloud compute config-ssh
does not populate the config with tpu-vms. This would be a good feature to have.The TPU's do not always start from the web console (giving an "unknown error"). It's better to do
$sudo reboot
.I notice that running libc.6.so gives a segfault instead of the version information like it is supposed to. This seems concerning to me, and I wonder if Julia's difficulties could be related.
rs@t1v-n-af474cc6-w-0:~$ /lib/x86_64-linux-gnu/libc-2.31.so
Segmentation fault (core dumped)
rs@t1v-n-af474cc6-w-0:~$ sha256sum /lib/x86_64-linux-gnu/libc-2.31.so
09d4dc50d7b31bca5fbbd60efebe4ce2ce698c46753a7f643337a303c58db541 /lib/x86_64-linux-gnu/libc-2.31.so
$ dmesg
[320933.001365] libc.so.6[1239142]: segfault at 15 ip 000056225fe0096d sp 00007ffeefde4d80 error 4 in libc-2.31.so[56225fd06000+178000]
[320933.001375] Code: 45 89 f4 48 39 fa 76 35 48 8b 05 b6 b4 0c 00 49 89 ff 64 48 8b 30 eb 0e 0f 1f 44 00 00 49 83 c7 01 49 39 d7 74 17 49 0f be 07 <f6> 44 46 01 20 75 ec 49 39 d7 72 8f 0f 1f 80 00 00 00 00 8b 7d 8c
valgrind report
...
==794602== ERROR SUMMARY: 4 errors from 4 contexts (suppressed: 0 from 0)
==794602==
==794602== 1 errors in context 1 of 4:
==794602== Invalid read of size 1
==794602== at 0x22796D: get_nprocs (getsysstats.c:178)
==794602== by 0x1F0838: posix_sysconf (sysconf.c:631)
==794602== by 0x1F0838: linux_sysconf (sysconf.c:106)
==794602== by 0x1F0838: sysconf (sysconf.c:36)
==794602== by 0x487ED81: GetSystemCPUsCount() (in /usr/lib/x86_64-linux-gnu/libtcmalloc.so.4.5.3)
==794602== by 0x485F95C: ??? (in /usr/lib/x86_64-linux-gnu/libtcmalloc.so.4.5.3)
==794602== by 0x4011B89: call_init.part.0 (dl-init.c:72)
==794602== by 0x4011C90: call_init (dl-init.c:30)
==794602== by 0x4011C90: _dl_init (dl-init.c:119)
==794602== by 0x4001139: ??? (in /usr/lib/x86_64-linux-gnu/ld-2.31.so)
==794602== Address 0x15 is not stack'd, malloc'd or (recently) free'd
...
I would need to download the symbols / source code to get more out of this.
Training v-diffusion-pytorch on TPUs
The training code for v-diffusion-pytorch is currently for training cc12m on tpus only. For dataset creation use your own or see dataset creation scripts for text and emoji dataset generation, and danbooru utility for face recognition and filtering on gwern's danbooru dataset.
You can download pretrained models for some of the experiments.
single character multiple font generation
Setup and Installation
Create TPU
Follow the quickstart guide to set up the gcloud cli. https://cloud.google.com/tpu/docs/users-guide-tpu-vm
cloud setup scripts has scripts for tpu creation and installation. Conda is recommended, but you can use whatever you prefer.
SSH and finish setup
Once you've created your tpu run:
gcloud alpha compute tpus tpu-vm describe generative2
acceleratorType: v3-8
apiVersion: V2_ALPHA1
`...`
externalIp: ...
`...`
state: READY
to get the ip address. I recommend setting an environment variable TPU_IP=...
for your shell, to keep commands portable when you are managing multiple tpus simultaneously.
Then you can transfer your project. (rsync, git or mounting a gdrive), and ssh in.
rsync -rvz --update --info=progress2 --exclude=*.pyc v-diffusion-pytorch USERNAME@$TPU_IP:/home/USERNAME/
gcloud alpha compute tpus tpu-vm ssh TPU_NAME
If you did not run the install scripts you will still need to set the following environment variables:
export XRT_TPU_CONFIG="localservice;0;localhost:51011"
export XLA_USE_BF16=1
And install the appropriate torch_xla for your torch:
pip3 install torch_xla[tpuvm] -f https://storage.googleapis.com/tpu-pytorch/wheels/tpuvm/torch_xla-1.10-cp38-cp38-linux_x86_64.whl
Install your project dependencies and you are ready to train.
Train
Start a training run, for instance:
python3 cc12m_1_cfg_train.py --train_set ~/datasets/drawtext/ --demo_prompts demo-prompts.txt --batchsize 3 --dataset_mode text --project_name drawtext-diffusion --max_epochs 100 --checkpoint checkpoints/cc12m_1_cfg.pth --lr 1e-5 --val_check_interval .5 --scheduler exponentiallr --gamma .99 --accumulate_grad_batches 8
You'll need to paste your wandb key the first time.
Configuration and CLI options
$ python3 cc12m_1_cfg_train.py -h
2022-02-27 04:32:51.146314: E tensorflow/core/framework/op_kernel.cc:1676] OpKernel ('op: "TPURoundRobin" device_type: "CPU"') for unknown op: TPURoundRobin
2022-02-27 04:32:51.146380: E tensorflow/core/framework/op_kernel.cc:1676] OpKernel ('op: "TpuHandleToProtoKey" device_type: "CPU"') for unknown op: TpuHandleToProtoKey
usage: cc12m_1_cfg_train.py [-h] --train_set TRAIN_SET [--val_set VAL_SET] [--test_set TEST_SET] --demo_prompts DEMO_PROMPTS [--checkpoint CHECKPOINT] [--batchsize BATCHSIZE]
[--scheduler_epochs SCHEDULER_EPOCHS] [--imgsize IMGSIZE] [--dataset_mode [{conceptual,drawtext,text,danbooru,goodbot}]] [--project_name PROJECT_NAME] [--lr LR]
[--gamma GAMMA] [--scheduler [{cosineannealingwarmrestarts,exponentiallr,onecyclelr}]] [--restore_train_state]
optional arguments:
-h, --help show this help message and exit
--train_set TRAIN_SET
the training set location
--val_set VAL_SET the val set location
--test_set TEST_SET the test set location
--demo_prompts DEMO_PROMPTS
the demo prompts
--checkpoint CHECKPOINT
load checkpoint file path
--batchsize BATCHSIZE
batchsize for training
--scheduler_epochs SCHEDULER_EPOCHS
epochs to pass to lr scheduler
--imgsize IMGSIZE Image size in pixels. Assumes square image
--dataset_mode [{conceptual,drawtext,text,danbooru,goodbot}]
choose dataset loader mode (default: drawtext)
--project_name PROJECT_NAME
project name for logging
--lr LR starting lr
--gamma GAMMA exponential decay gamma for lr
--scheduler [{cosineannealingwarmrestarts,exponentiallr,onecyclelr}]
choose dataset loader mode (default: None)
--restore_train_state
restore lightning training state
You can ignore the TPURoundRobin
and TpuHandleToProtoKey
warnings.
If you do not pass a val_set it will split the train_set into a train and val set for you.
In addition to the explicit args you can pass all the pytorch lightning Trainer parameters. For example accumulate_grad_batches
or fast_dev_run
Dataloaders
v-diffusion-pytorch includes a few dataloaders for various experiments. They are substantually the same, generally just loading labels from json, but may be useful for loading your own datasets.
Caveats
This is fairly hacky and doubtless has bugs. Currently trying to load a checkpoint while using CosineAnnealingWarmRestarts crashes since the
initial_lr
is not set.Lightning tuner and profiler does not work.
This is formatted with black.
Acknowledgements
Thanks to Katherine Crowson for the original code, and thanks to TPU Research Cloud for the tpu credits!
Generating Python Code with Transformers
2021-03-10
In Karpathy's famous essay The Unreasonable Effectiveness of Recurrent Neural Networks his LSTM based network generates C code after training on the Linux source. This demonstration was sufficiently impressive that every now and then I'll revisit how well current language models can generate code.
For this I scraped python code from github and trained on a variety of transformers based networks. It looks pretty good:
def find_match(pathname):
# -- Newline characters help
try:
newline_line = dirname.encode('utf-8'))
except UnicodeError as ex:
raise AssertionError('Invalid option "%s" for separator. '
'pathname must be an ASCII string')
# This is the first time this checks a Windows prompt.
# On Windows, if you don't have any nested types in start_when, the
# *option* is not stored.
newline_line = str(str(str(ex))[1])[0]
if newline_line is None:
return None
self._add_option(newline_line)
def _from_option_vars(self):
# -- Default command for all command line arguments
return self.line_argument_group.args
def _get_number(self):
# -- Mark as variable name for how to call the argument.
return self.int_argument_group[::1]
def _get_control_title(self):
# -- Controls a char string for horizontal content
for command in self.command_list:
if command in getchar_string(command):
continue
if getchar_string(command):
command.title = command
return command
Danbooru Utility
2021-03-09
I made Danbooru Utility to make working with gwern's Danbooru20XX dataset easy.
It can explore the dataset, filter by tags, rating, and score, detect faces, and resize the images. I've been using it to make datasets for gan training.
Problem Solving with Deep Q-Learning at IEEE RoboResearch
2016-06-01 10:00
Download the slides here, or with:
git clone https://github.com/reidsanders/dl-talk.git
Follow along on the slides as the video runs:
Deep Learning Talk at the Triangle IEEE Robotics and Automation group
2016-02-05
Download the slides with
git clone https://github.com/reidsanders/dl-talk.git
Follow along on the slides as the video runs:
I glossed over a lot by necessity. I hope if you are interested you will try it yourself by following a tutorial or running an existing project.
If it was me, I'd start with:
Implementing a Neural Network from Scratch.
If that appealed, I'd continue on with the tutorials on that blog. If I wanted something with more breadth, I'd go to:
If I wanted to learn machine learning from the ground up, I'd go here:
Stanford Machine Learning Course
UFLDL Tutorial: A Matlab/Octave, fairly math heavy tutorial.
For Deep Learning in depth, I'd go here:
Neural Networks and Deep Learning: a more in depth textbook.
A great community:
A good source of new datasets:
Why has someone been paying 100x market price for GPU instances?
2015-07-24
For the past 10 weeks EC2 GPU spot instances on US East have been going for 15-100x the price in other regions.
I've been using Amazon's GPU instances for running deep neural network's, and have been quite impressed by the ease and cost. Spot bids, where your instance is terminated if the bid price goes above your maximum bid, often go for 5-10x less than on demand instances. Recent advances in deep learning have relied upon large neural networks using high end GPU's, but getting your own hardware is expensive, and a big lock in. GPU instances are quite affordable, scalable, and the spot bid prices reliable enough to minimize the risk of having a training run cut short.
One mystery struck me when I was looking at for the best region to run these instances on. Who is spending 100x the going spot price on g2.2xlarge instances in US East?
Beginning on May 7, and ending on May 29, the spot price for g2.2xlarge instances in us-east-1e was $6.00.
When I restricted my bid to other availability zones.
aws ec2 request-spot-instances --spot-price 0.50 --launch-specification "{\"KeyName\": \"my-key\", \"SecurityGroups\": [\"myip\"],\"ImageId\": \"ami-0f53a04b\",\"InstanceType\": \"g2.2xlarge\", \"Placement\":{\"AvailabilityZone\":\"us-east-1a\"}}"
I found they had no instances at all.
Compare this to the going price of $0.065 in us-west-1, and other regions. On May 29, the price dropped to $2.60, still twenty times the rate in us-west-1. On the twenty-third of July, this price briefly spiked back up to $5.00, then dropped to the price of on demand instances, $0.65.
Why would anyone pay ten times the on demand rate, and one hundred times the spot bid price? On demand instances would be much more reliable, as Amazon can drop your spot instance whenever it wants. Moreover, this customer is taking the entire available supply for US East, Amazon's largest region. Such a huge customer would also be able to make a long term deal for a lower rate.
Looking at these charts, you might notice occasional spikes to $5.00 or $6.00. As this forum thread indicates, these price spikes have been going on for a while. Apparently this is caused by companies that don't want to lose their instance, and are willing to occasionally pay significantly more than the market rate for a long term cheaper solution. Sometimes the spot supply shrinks, and all that is left is a few high end bidders. In many cases they would have saved money, but a spike lasting ten weeks makes this strategy financially inefficient. So why did this happen? Here's a few theories.
Some company or government set the price way higher than they expected the price to ever reach
(>=$6.00)
to prevent being outbid, and losing their instance.Then someone else did the same thing
(=$6.00)
.They accidentally bid against themselves.
They simply didn't notice the price spike never went down, and have been paying enormous prices for months.
The spot instance availability for this entire time period dropped so much that there are only a few instances being paid for. Still unnecessarily expensive, but if they are running something that can't be interrupted, it might make sense. I still recommend not running critical, month long operations on spot instances.
Someone thought they were bidding in cents, not dollars.
Actually, they are so price insensitive they don't care about expanding to other regions, using on demand, reserved, or g2.8xlarge instances, or buying their own hardware.
It's something Amazon is doing.
Maybe Amazon sources on demand instances from the spot pool by simply bidding very high. This would be an unusual hack, but it's not unthinkable.
Maybe Amazon doesn't actually have any g2.2xlarge instances in the US East spot pool. They did have them in the on demand pool.
It's a bug.
If you have any insight, let me know. I'm asking Amazon on twitter, and I'll update if I learn something new.